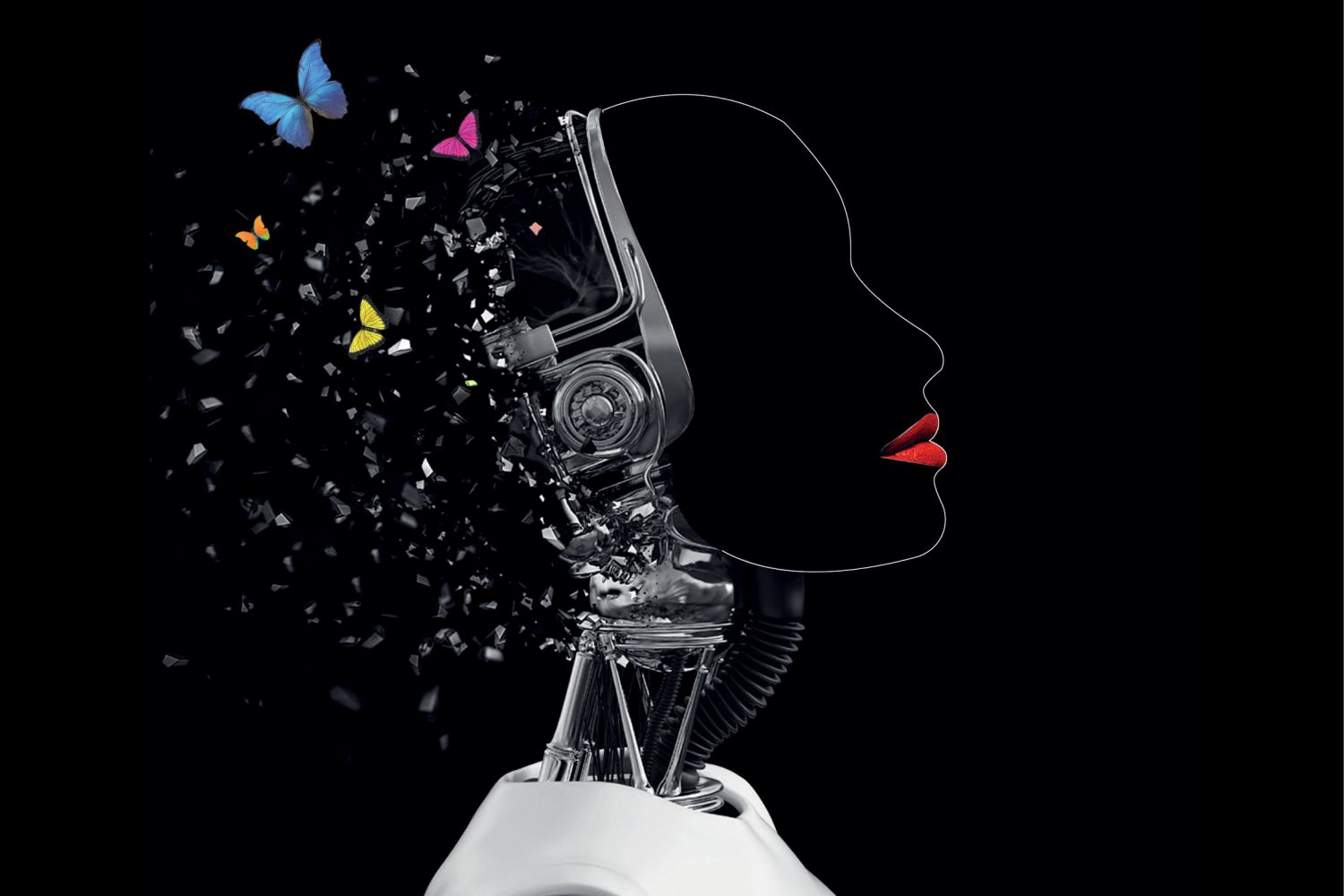
I picked up
my smart phone and clicked on Wysa, “the compassionate AI chatbot for
behavioural health”. Up popped a
cutesy little penguin: “Hi, I’m Wysa,” it said, “I’ll help you manage stress
and stay positive.”
AJ: “I’m working on a very stressful
article on artificial intelligence.”
Wysa: Oh
okay…Could I do a quick check for how stress might be affecting you?
I guess.
Over the
last two weeks have you had any of the following problems…
Had tro